Data de-identification is the process of removing or altering personally identifiable information (PII) from datasets in order to protect the privacy of individuals.
In the world of software development, engineering teams have a responsibility to limit access to that data, often due to regulations that dictate how PII can be used. (Spoiler alert: software development is not a reasonable or acceptable use.) However, real-world data like PII contains all of the nuance, intricacies, and random edge cases that developers need in order to properly develop and test the applications their users rely on.
Data de-identification is one way to keep private information safe while still getting developers realistic data that captures real-world complexity.
In this guide, we will define data de-identification, call out some key techniques for de-identifying data, and look toward future trends in data privacy.
By way of example, we’ll consider a hypothetical Starbucks rewards program and the app Starbucks uses to offer the program to consumers, which, of course, runs on its consumers’ data. Maybe even your data. If you’re a part of the Starbucks rewards program, their database has a plethora of your personal data—your name, address, birthday, credit card numbers, etc. Starbucks also has an estimated 1,500 software engineers. You see where we’re going here. Read on to see how data de-identification comes into play.
Defining data de-identification
Data de-identification is any action taken to eliminate or modify personally identifiable information (PII) and sensitive personal data within datasets to safeguard individuals' privacy. By de-identifying data, organizations can minimize the risk of unauthorized access to sensitive information and avoid the legal consequences of infringing regulations like GDPR, which can involve hefty fines.
Often, the motivation for de-identifying data is to provide realistic data for lower environments, such as development, testing, and staging environments. One method of data de-identification is redaction—the infamous black bar or XXXXs. Redaction is very secure, of course, but it also strips the data of its utility.
At the heart of the question of how to best de-identify data is the inherent tug of war between how much privacy and how much utility you want to preserve. As we consider the different approaches, we’ll keep this tug of war in mind and address how each approach leans more or less one way or the other.
Methods of data de-identification
There are a number of high-level methods to effectively de-identify data, and within each of these high-level methods is an array of more detailed techniques that can introduce variability in the quality of the output data you achieve. The type of data you are working with as well as the format it is stored in will help you determine the most effective method to de-identify your data. Below is a summary of some of the more common methods.
Redaction removes or obscures sensitive information. Its close cousin suppression, meanwhile, completely omits the impacted data from the dataset. While generally easy to implement and very secure, you lose all of the data’s utility. You don’t know if one person ordered a million mocha frappuccinos or if one million people ordered one mocha frappuccino. Generally, having some distinction between individual users is important to allow for identifying and working with different use cases and scenarios.
Aggregation / Generalization : These approaches include reducing the specificity of the data by aggregating it (mean, median, etc.) or putting it into a more broad format like ranges (generalization). For testing, you lose the vantage point of edge cases and outliers through aggregation. In addition to making the data less useful, generalization can also fail to properly obscure PII due to quasi-identifiers persisting in the dataset.
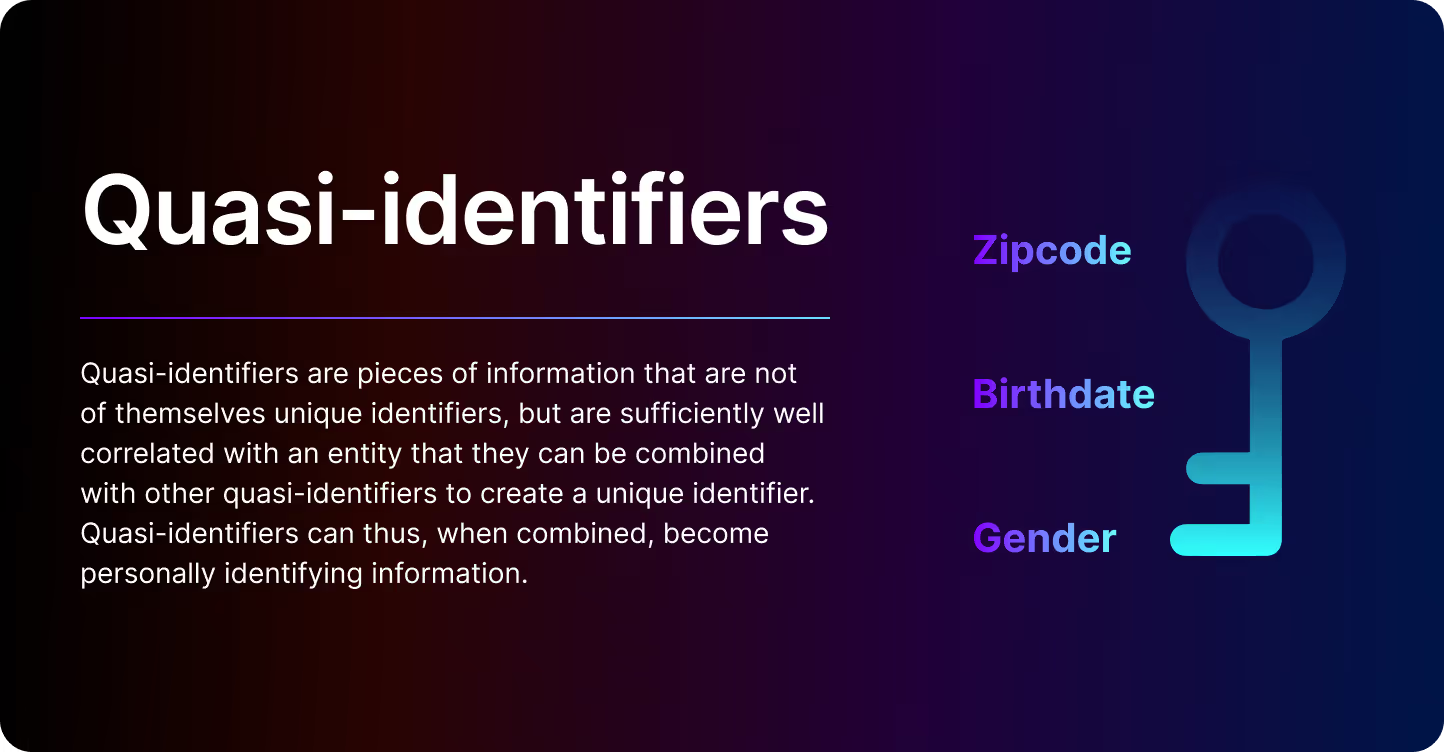
While you can try to balance the utility of your output against privacy by adjusting k-anonymity, l-diversity, and t-closeness, those considerations and design decisions make these approaches much more complex to implement. Ultimately, it requires a lot of foresight and understanding of your data to know exactly how to set your buckets for generalization or aggregation.
Subsampling, or subsetting, is selecting a random or representative sample of your data rather than using the entire dataset. While it does help to minimize your dataset, protecting the majority of data within it, any individuals whose records are within the subset are left fully exposed. You’d know that Robert always gets a Venti Java Chip Frappuccino with light whip and an extra shot of espresso.
Subsetting on its own is not a data de-identification method; it is purely a data minimization method. It can also be quite difficult to subset across a full database while keeping your output small since pulling records in from referenced tables can pull more records, ballooning the size of your final dataset.
Masking, often used interchangeably with the term data obfuscation, broadly refers to protecting sensitive data by replacing it with a non-sensitive substitute. Some techniques include pseudonymization, anonymization, and scrambling. While some techniques are better than others, they are all generally aiming to give you the most useful data while maintaining privacy.
Challenges of data de-identification
There are a number of challenges inherent to building and maintaining a process for data de-identification, including the initial configuration of your methods of de-identification, changes to your data over time which require ongoing maintenance, ensuring adequate privacy protection, and achieving data utility in your output data.
1. Difficult to set up
If our database was a simple table with just name, email, and order, it would be easy to set up. However, today’s data is complex, multi-dimensional, and distributed across multiple, divergent sources (app is built on Postgres but payments are on Oracle). Combine this with evolving regulatory requirements and you have a taller than expected mountain to climb. Both the homegrown scripts and legacy tooling that organizations once relied on to de-identify their data are no longer living up to the task.
2. Costs to maintain
As your database changes, so do your inputs and how you choose to de-identify your data will continue to impact your process. You’ve coupled the evolution of your database with the need to update your de-identification solution. Every time you change your production schema, you'll need to make sure that you're adapting your approach to de-identification to handle any new data correctly. This is why we see drift between production environments and lower environments, because the cost of maintaining can be quite onerous.
3. Sufficient privacy
If you are going to use aggregation or generalization, we’ve alluded to the fact that you might actually be insufficiently de-identifying your data. It is very possible that given a set of quasi-identifiers, you might be able to piece together or reconstruct who a given person is in your dataset. To avoid this requires significant upfront work to understand your data and understand what your k value needs to be to ensure sufficient privacy.
4. Sufficient utility
Too often, once you achieve sufficient privacy, you’ll discover that your data is no longer useful. If you are using aggregation, you may find that the final resulting buckets are not actually useful, making aggregation functionally equivalent to pure redaction. Additionally, you want specific cases in your data that aren't aggregated to test for precise input use cases. Insufficient utility can also rear its head with poorly masked data. If the data doesn’t take relationships into account or is inconsistently masked across tables, you can’t use the whole database—which defeats the purpose of trying to have useful test data.
Legal and ethical considerations
Increasingly, organizations are required to perform data de-identification to adhere to legal and ethical guidelines. Often an organization will have a data privacy policy that engineering teams must adhere to during development.
At minimum, if you work with regulated data, it is crucial to ensure compliance with data protection regulations such as GDPR and HIPAA, to protect individuals' privacy rights. These policies are written to ensure the protection of individuals' most sensitive information, such as healthcare identification in the case of HIPAA.
Adhering to data privacy regulations might also involve maintaining transparency by informing individuals about the data de-identification processes used. It is a significant ethical consideration to inform individuals about what their data is being used for and how the organization they are volunteering it to is protecting it. This makes it that much more important to have reliable data de-identification tools in place.
Accelerate your release cycles and eliminate bugs in production with realistic, compliant data de-identification.
Real-world applications of data de-identification
A broad range of industries collect data for a variety of use cases that can each require their own flavor of and approach to data de-identification:
These are just a few examples of industries in which data de-identification is essential, both for software development and for other use cases. The truth is, data security should be on your mind no matter what industry you find yourself in and data de-identification is a great first line of defense.
Future trends in data privacy
One of the challenges in data de-identification is the changing requirements of data privacy legislation combined with all of the ways that data is changing in the world. As technology continues to advance, the need for organizations and individuals to remain vigilant and adaptable in the face of evolving privacy challenges will become ever more essential. Here are some of the trends that we see
At the end of the day, by employing effective de-identification methods, organizations can balance data usability and privacy, ensuring compliance with legal and ethical standards. Data de-identification is a powerful solution to the complex problem of data privacy and security.
Selecting the right data de-identification method
You may be wondering which data de-identification method is the best or the right way. The answer is one that no one likes to hear: it depends.
Many use cases are simple enough that the speed of redaction or suppression is the most viable option. However, as the use case becomes more complicated or nuanced, data masking will be the most useful for engineering teams. Even with that, there are different methods of data masking (link to data masking guide) - each with their own ideal use case. Regardless of which way you choose to mask, creating the infrastructure from scratch requires extensive setup and ongoing maintenance. Luckily, out-of-the-box solutions exist to save you the headaches.
Enter Tonic Structural
Tonic.ai exists to provide easy setup and maintenance of data de-identification workflows so that developer teams can reap the benefits of having useful de-identified data to accelerate their engineering velocity.
Tonic Structural integrates the latest methods of data de-identification, synthesis, and subsetting to provide organizations peace of mind as they use their data in software development and testing. With rapid access to quality test data that captures all the nuance of production, without putting sensitive data at risk, developers accelerate their release cycles, catch more bugs, and ship better products faster. To learn more about Tonic Structural’s success stories, check out our case studies, with examples in financial services, healthcare, e-commerce, and more.