Expert insights on synthetic data
The lastest
Deep dive: small vs large language models for token classification
Check out this talk from Ander Steele, Head of AI at Tonic.ai, which was first presented at ODSC East in Boston, and explores the evolving landscape of named entity recognition (NER); specifically comparing the performance of small language models versus large language models for token classification tasks.
%201.png)
Blog posts
Thank you! Your submission has been received!
Oops! Something went wrong while submitting the form.
Technical deep dive
Data de-identification
Generative AI
Tonic Textual
Data de-identification
Generative AI
Tonic Textual
Data de-identification
Test data management
Data privacy
Tonic Structural
Tonic Textual
Product updates
Tonic Ephemeral
Tonic Structural
Tonic Textual
Tonic Fabricate
Product updates
Data privacy
Tonic Textual
Test data management
Data privacy
Tonic Structural
Tonic Textual
Tonic Ephemeral
Data de-identification
Data privacy
Generative AI
Healthcare
Tonic Structural
Tonic Textual
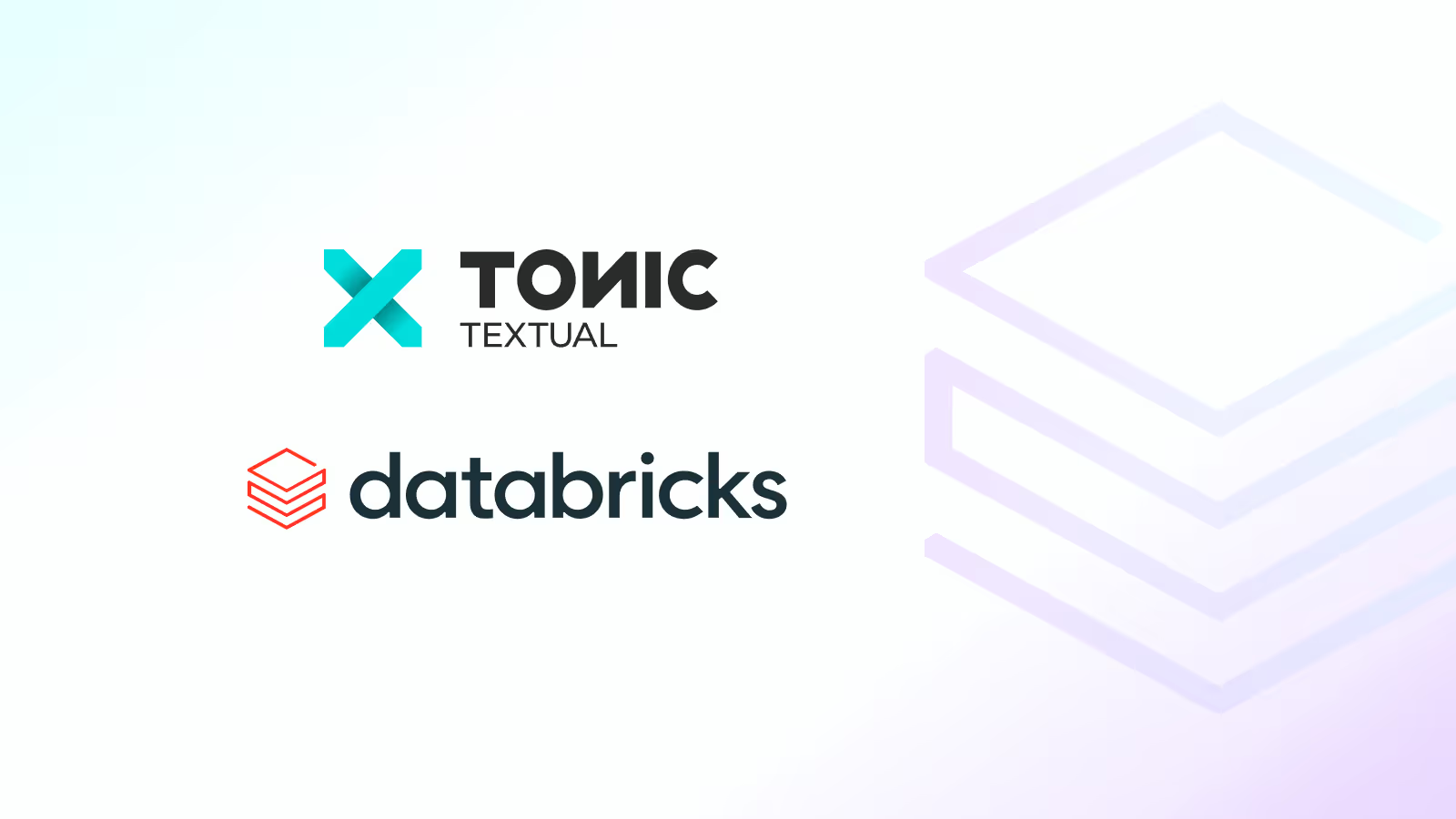
Tonic Textual is now on the Databricks Marketplace: unstructured data, meet easy ingestion
Product updates
Generative AI
Product updates
No items found.
Data privacy
Generative AI
Healthcare
Data de-identification
Tonic Structural
Tonic Textual
Tonic.ai editorial
Product updates
Tonic Ephemeral
Tonic Structural
Tonic Textual
Tonic Validate
Technical deep dive
Tonic Structural
Tonic Fabricate
Build better and faster with quality test data today.
Unblock data access, turbocharge development, and respect data privacy as a human right.
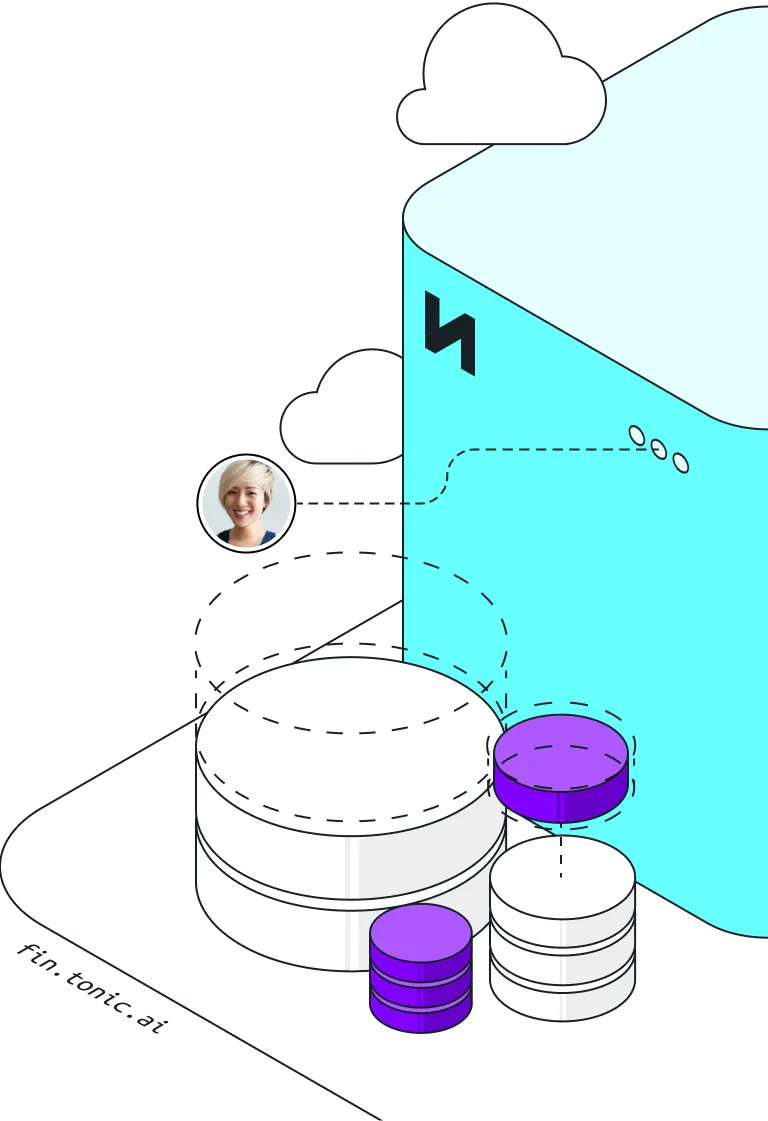